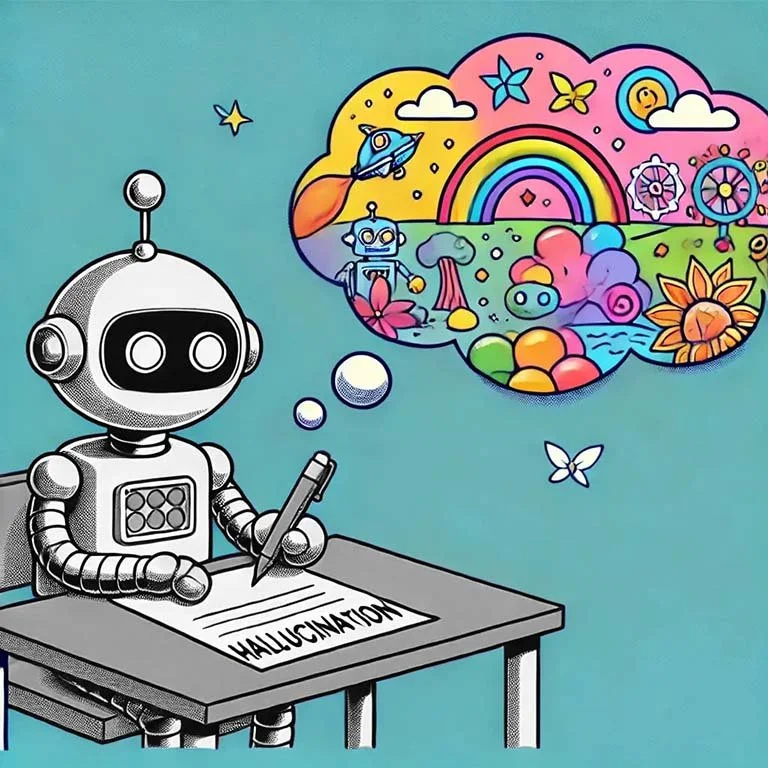
Hallucinations, Fact checking, Entailment and all that. What does it all mean?
One of the big problems in AI is that the systems often hallucinate. What does that mean exactly and how do we mitigate this problem, especially for RAG systems?
One of the big problems in AI is that the systems often hallucinate. What does that mean exactly and how do we mitigate this problem, especially for RAG systems?